Century Old Mystery of The Zipf's Law (And A Cool Experiment)
Yes that's not a typo at the title, there is something called the Zipf's law, and it's quite .. weird.
In fact, in its origins, it is a law pertaining linguistic patterns used in languages, and it governs how often do we use specific words and their natural frequency of occurrence.
And the reason of its mystery, is due to its surprising patterns that not only apply to English language, but essentially to any other language, including "Esperanto" (in case you did not know about Esperanto, it's a constructed language that goes back to 1887 - I didn't either till a month ago, when some fun guy was conversing with it on steemit and I learned about it, google translate even supports it! For more info on it, check out this wikipedia article).
And back to topic...
Yet while this will probably get a bit lengthy - don't forget the experiment at the end! :)
What is Zipf's Law?
The Of And To A, In Is I That, It For You Was, With On As Have, But Be They
No I did not go on a weird rant with that sentence above, nor did I lose my sentence-creating grammatical skills all of a sudden. In fact, that sentence above represents the most widely used words, in order, of the English language. Commas are pointless, but just to confuse you a bit lol
And that concept is at the core of Zipf's law.
In fact, the law identifies that the frequency of a word's usage in the studied context is inversely proportionate to its rank.
This applies into any context in which the words are considered. Whether it's the whole English literature, Shakespeare's work, a book, or even this article.
So for instance, the second most ranked word would appear half the times of the most used word. The third most ranked would appear a third of the top used word. See Image below for a sample chart
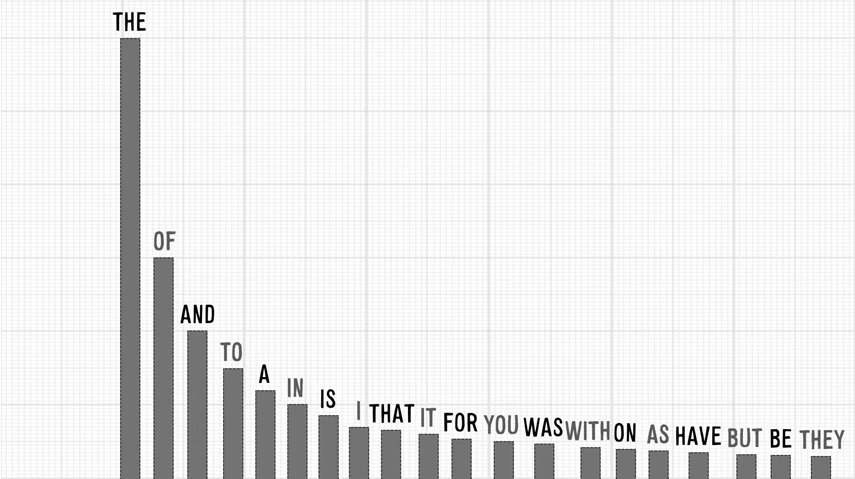
Chart showing the rank vs frequency of words appearance
Let's take a more concrete example. A study on the Brown Corpus of the American English found that the word "the" (similarly to our weird sentence above) is the most used word, at 7% of the time (69,971 out of around 1 million words). The second most used word was "at", at around 3.5% of the time (36,411 appearances), and then "and" at close to 2.34% (28,852 appearances). More examples in the graphs below.
Most often, the law and its charts are plotted as log value points of (frequency - rank), such as the below, which shows results based upon ranking words and frequencies across 30 wikipedias' first 10 million words
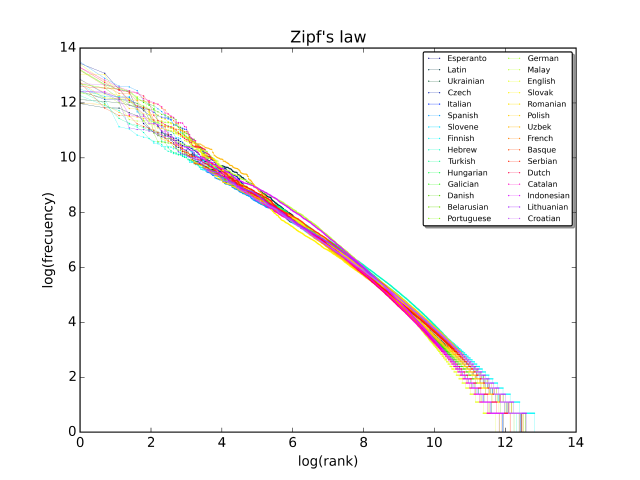
Plot of rank v/s frequency for first 10 million words across 30 Wikipedias
Not even Shakespeare, Dickens,... escape the Zipf's Law! Check out below chart.
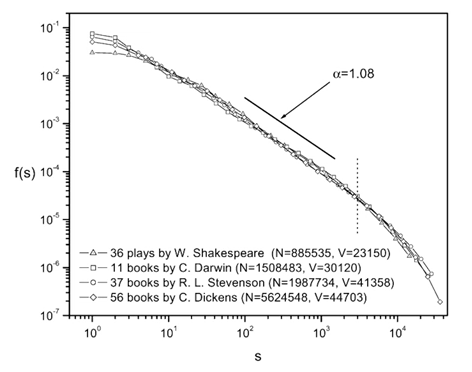
Chart of frequency - rank within the works of different English Literature authors
Brief History
The Law is actually named after American linguist George Kingsley Zipf, who even though was not the first to identify its occurrence (prior separate works were done by Jean-Baptiste Estoup, and also Felix Auerbach around 1913), yet he was the first to popularize it and try to explain it between 1935 and 1949.
Can it get weirder?
Zipf's law appears to apply everywhere! City population ranks across countries, website traffics, last names, number of phone calls received, income rankings, corporation sizes, ... even rates at which we forget !
The law itself is considered a somewhat discrete form of the pareto distribution (see more info about Pareto Distribution here), which leads to the Pareto Principle.
The latter is actually what dictates the famous 20-80 principle,
i.e., 20% of the causes are responsible for 80% of the outcome
such as:
- 20% of the population has 80% of the riches
- 20% of the words account for 80% of the texts.
- 20% of your customers, account for 80% of your profit.
Explanation?
Well, the reason we just called it mystery, is because, as of yet, there is no well understood explanation as to why it occurs.
Several attempts have been made to explain it. Begining with Zipf himself, who generally attributed this to the "principle of least effort".
Whereby his theory was that people attempt to utilize lesser words naturally to explain themselves, since it's well, easier. Yet listeners needed more larger vocabulary to provide better specifics to further understand, so as their task becomes, easier too. And hence it is the compromise between speaking and listening is what results in few words being used more often, and the most words being used infrequently.
Other recent studies along the same lines considered that the use of short words frequently helps minimize the load on the listener's processing.
Later research(works of Wentian Li and then Benoit Mandelbrot) yet suggested that this is a very normal and mathematical common way for sentences and even random texts to appear. They even suggested that typing random words on a keyboard without any sense would yield similar results as to Zipf's law, due to the probability of shorter lettered words to occur more frequently than others. Yet, the caveat with this approach is that natural language is deterministic, and is due to factual data and information being conveyed, which negates the fact that it would apply to a random set and based on simple probabilistic formula. Point in case is that this approach fails to explain how names of months, planets, or even chemical elements follow zipf's law distribution. See photo below showcasing said distribution
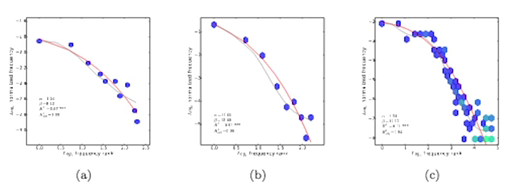
Zipf's law applying to a: months, b: planets, c: elements occurrences
Another reasoning has linked Zipf's law to Preferrential Attachment, which can simply be explained in the rich get richer, the successful get more successful. So a word getting used often, will get used more often. The more views a video gets, the more likely it is to get recommended. The more views a post (steemit post, maybe lol) gets, the more upvotes it could get.
Own Experiment with this text
So I decided to run a cool Zipf's experiment with this text to see how it ranks. I've used this free site for counting the words: https://wordcounter.com/
And then my results came as follows:
Plotting in onto a chart also gave the following:
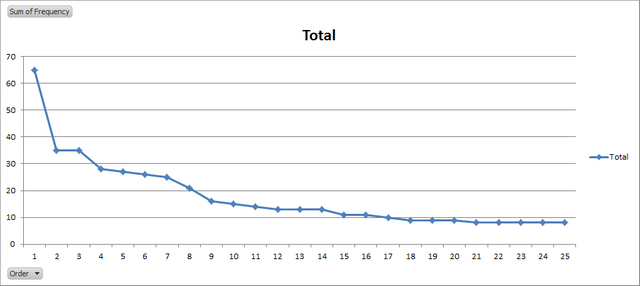
So there you have it .. a Zipf, with "the" most used, and the typical words being the most frequent too :)
Hey, your turn! you might want to do your own experiment with your own text or any of your own posts, and show me your top ranking 5 words and their count!
References:
Photo Credits:
Founder of Arab Steem
Arab Steem is a community project to expand Steemit to the Arab world, by supporting the existing Arab steemians and promoting others to join.
You can connect with us on @arabsteem or via discord channel https://discord.gg/g98z2Ya
Your support is well appreciated!
Proud Member Of
- steemSTEM: SteemSTEM is a project that aims to increase both the quality as well as visibility of Science, Technology, Engineering and Mathematics (and Health). You can check out some great scientific articles via visiting the project tag #steemSTEM , project page @steemstem, or connecting with us on chat https://steemit.chat/channel/steemSTEM
- MAP(Minnows Accelerator Project): MAP is a growing community helping talented minnows accelerate their growth on Steemit.
To join, check out the link at the home page of @accelerator account
Check our some of my Prior Posts
- Day 7 of my #sevendaybnwchallenge photography
- Day 6 of my #sevendaybnwchallenge photography
- Auriez-Vous Avaler Une Batterie?
- Day 5 of my #sevendaybnwchallenge photography
- Since Bigger is Better: Meet UY Scuti
- Day 4 of my #sevendaybnwchallenge photography
- MRI Scans and How They Work
- Day 3 of my #sevendaybnwchallenge photography
- Pinktober: Breast Cancer Awareness
- Day 2 of my #sevendaybnwchallenge photography
This is so cool!
It could lead one to believe we ARE in a matrix. 😵 I mean, everything always seems to work out perfectly mathematically.
Can you explain what you mean by the months, planets, etc. also having this quality? The pics are too tiny for me to see what's going on in the graph.
I understand plotting the frequency of word but don't quite know how OTHER things are plotted using this.
It's a frequency thing... but what 'frequency' is measured in the planets to come to this conclusion?
Regarding elements. Is that how often they appear in nature compared to the next?
Thanks for being so smart, man. I really appreciate your posts.
haha yea thats always a possibility
about the months, planets,... it refers to their names occurrences also within texts, like how frequently they would show in the text compared to their ranks, which kinda defies mathematical randomness. Sorry couldn't find a higher resolution image.
For the plotting, it is just the frequency of occurrence vs the rank (which is the top, second, and so on and so forth...), other graphs are using logarithmic function of said frequency for plotting too
For occurrence in nature/elements, apparently zipf's law can correlate to tons of things, this could be an aspect.
Awww and thank you for being so awesome !
I think a Zipf analysis of word counts for a language could be useful when attempting to implement an artificial intelligence with semantic meaning. The Zipf distribution gives an insight into what a particular culture thinks are its most important words/concepts, as viewed by the culture using that language.
For example, many languages have a particular word that represents the concept of a "wall." However, at least one that I know of uses two different words to distinguish from "inside wall" and "outside wall." For the culture using that language, the distinction was important, for some reason.
Language is more than a means of communication. It provides a way of thinking and solving problems, especially those problems that require more than one individual to solve.
Let's look at your first list of important words, by decreasing frequency: The Of And To A, In Is I That, It For You Was, With On As Have, But Be They. From this I would hypothesize that this culture (the one using English words) collectively thinks that it is very important to represent the distinction between the definite ("the") and the indefinite ("a," "an). So if I were to implement an AI that actually understood semantics, I would want it to learn the following as general concepts:
Current state-of-the-art cannot do this. Hinton thought vectors (from NLP research) tend to group words in close "proximity" within the network based upon whether statistical usage permits one word to be substituted for another, such that the result is still a sentence that has "some" meaning. But there is no guarantee that the result of the substitution has the same meaning.
Thank you for the insights. Yes your take on this could open interesting doors, particularly with the crazy amount of AI progress we are having now.
It might already be in the works somewhere, but if not, you might have an amazing idea to proceed with.
Thanks again!
You're welcome, and thanks for the kind response. I really enjoyed reading your article. It was very well written. I almost forgot ... I wanted to throw @originalworks at this to snag another upvote for you. ;)
Wow! That is crazy!
Every time I read one of your post I learned something very interesting thank you for sharing and keep up the great work You have my vote
Glad to hear that, Manny, thank you!
This is very interesting did not know about it either.
Thank you bigbear ! :)
This post has received a 0.52 % upvote from @buildawhale thanks to: @mcfarhat. Send at least 1 SBD to @buildawhale with a post link in the memo field for a portion of the next vote.
To support our daily curation initiative, please vote on my owner, @themarkymark, as a Steem Witness
Congratulations! This post has been upvoted from the communal account, @minnowsupport, by mcfarhat from the Minnow Support Project. It's a witness project run by aggroed, ausbitbank, teamsteem, theprophet0, someguy123, neoxian, followbtcnews/crimsonclad, and netuoso. The goal is to help Steemit grow by supporting Minnows and creating a social network. Please find us in the Peace, Abundance, and Liberty Network (PALnet) Discord Channel. It's a completely public and open space to all members of the Steemit community who voluntarily choose to be there.
very nice
great post
thank you