Answering 4 RG Questions related to PCA
Last week I was checking Alexa and realized that there are 1.070 backlinks to Steemit. Not bad, but still not all that much that it's impossible to make a difference.
There was something else, also encouraging. Although we all brag about the content on Steemit, the majority of traffic is actually - organic. "Bouncing rate" is fine as well.
So let's bring more traffic by targeting specific niches.
It could be useful in general and particularly for our STEM community.
Questions:
- Should you implement correlation before or after factor analysis? (link)
- What is your suggested solution, when the correlation matrix is not positive definite? (link)
- How can we interpret negative factor loading? (link)
- Factor Analysis: Which method and rotation should I use? (link)

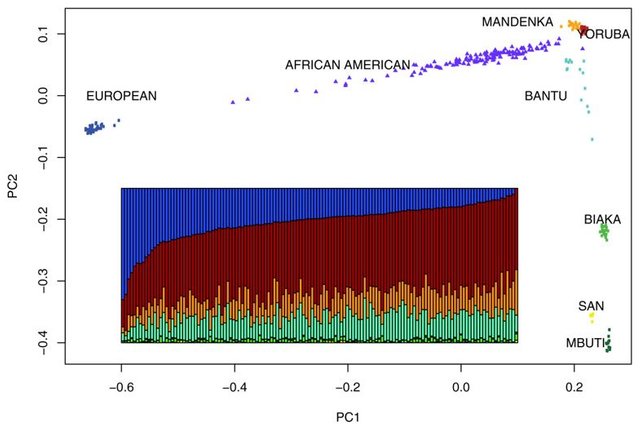
Should you implement correlation before or after factor analysis?
After you pass your 20th dataset, you will develop some sort of intuition, what is working well and what is not working - at all.
It's useful to construct correlation matrix before you begin.
It could happen that one of the components is the complete "outgroup" and that "column" / "row" will affect the result by "compressing" actually relevant data to the unsolvable cluster.
If that is the case, ok, do PCA with all the data. Remove those problematic. And run the analysis again!
Check the link that I gave you and see the difference
Another scenario could be that you will find several groups of data, strongly correlated within the group and very distinctive from other groups.
If you use the "whole dataset", you will fine - those groups!
If you want to look deeper, analyse those group separately.
What is your suggested solution, when the correlation matrix is not positive definite?
By the definition that I've found here:
> A matrix which fails this test is "not positive definite." If the determinant of the matrix is exactly zero, then the matrix is "singular."
In practice:
The solution is simple, delete :D
How can we interpret negative factor loading
Factor loading corresponds to correlation coefficient. If it's negative - you have negative correlation. From the example I gave you, European vs African if you are reading alongside PC-1 and if you read alongside PC-2, what do you see?
There are cases when you strictly need to extract only positive values.
For example, fluorescence is additive, every component is contributing in positive manner... In that case, you can't use PCA. You need NMF for example.
Factor Analysis: Which method and rotation should I use?
This one is difficult, both from "phylosophical" and from practical perspective.
If you know what you should expect, in spectroscopy for example - it's easy.
Acept only those values with physical meaning.
If you don't know what to expect... God mercy on your soul... The problem is:
result = compoent x coefficient + error
If your componets are wrong - your coefficients are wrong as well! You are doomed :D
There is a rule of thumb:
do you have any column full of zeros or some NaN?
do you have missing values
do you have the whole column with the same values (usually zeros or too high values, saturation...)
do you have a completely linear change which is exactly the same (example: 1 2 5 7 and 10 20 50 70)
- try without rotation
- try with orthogonal rotation
- try with oblique rotation
For the majority of datasets, all three solutions will be very similar. Use the first one, avoid questions "why were you complicating for no reason?"
If the components are independent(ish), the second solution will be different from the first and the third one will be almost the same as the second. Use the second one
If the components are very similar, the third option will be very different from the second one. Usually in spectroscopy, especially fluorescence spectroscopy, use the third one
JOIN STEEMIT, BLOGGING PLATFORM, AND EARN CRYPTO!
This post has been voted on by the SteemSTEM curation team and voting trail in collaboration with @utopian-io.
If you appreciate the work we are doing then consider voting both projects for witness by selecting stem.witness and utopian-io!
For additional information please join us on the SteemSTEM discord and to get to know the rest of the community!
To listen to the audio version of this article click on the play image.
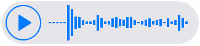
Brought to you by @tts. If you find it useful please consider upvoting this reply.