#8 Translation Category Part 3 - What Metrics Do Professionals Use To Evaluate Translation?
In this article, we want to concentrate on the metrics by which professionals use to evaluate the quality of a translation work. We are now dealing specifically on the translation text.
These metrics are measurable as they use a points-based system to measure quality objectively. Points are given to errors made in the translation. The more serious the error, the higher the points. Therefore, the less points a translation scores, the higher the quality in the translation.
The errors are usually divided into 7 categories and each category is outlined comprehensively depending on the metric system used:
- Wrong Term
- Wrong Meaning
- Omission
- Structural Error
- Misspelling
- Punctuation Error
- Miscellaneous Error
A quality translation text free from error should fulfill:
- Correct information is translated from the source text to the target text.
- Appropriate choice of terminology, vocabulary, idiom, and register in the target language.
- Appropriate use of grammar, spelling, punctuation, and syntax in the target language.
- Accurate transfer of dates, names, and figures in the target language.
- Appropriate style of text in the target language.
A high quality of translation should be completely accurate and totally error free.
In my research, I discover that there are 4 different kinds of metrics companies can use to evaluate the translation text objectively. The metric system of each one is quite extensive, so I am summarizing the main points of each metric so that we can get an overview of how the mechanics work for each one. When errors are made in translations, each metric has its own criteria to assign points according to the severity of errors made.
1. LISA QA metric
The LISA Quality Assurance (QA) Model was developed in 1995 and distributed by the Localization Industry Standards Association (LISA) for localization projects.
The Lisa method sets some specfic metrics for quality control of translations. It is a mechanism formulated to take out the subjectivity in the evaluation such that the quality assurance process is evaluated objectively. To do so, some statistical information needs to be addressed to define the quality metric.
The importance of LISA is pointed out from this article source:
How important is it? The LISA (Localization Industry Standards Association) method aims to maintain the quality and accuracy of the review process. Reviewing text is of fundamental importance in seeking the best possible quality for translations. Various issues are taken into consideration during the review, such as grammar, terminology and terminological appropriateness. During the review, it is also possible to assess whether the text is in agreement with the standards required by the customer. The LISA method serves to organize these issues and standardize the method of evaluating the translation, providing an overview of the quality of the translated text. The LISA method also functions as a feedback tool for the translator: the translator receives a report with the errors and/or improvements indicated during the review, helping to improve performance in future jobs while promoting continual follow-up.
Here is how LISA QA works in summary form:
- It evaluates the translation on a point system
- The maximum number of error is based on the number of words in the translation text
- 3 minor errors in a 200 word translation would mean that the translation is in LOW quality thus deserving failure
- Only 2 errors are allowed to pass the quality of translation
Areas of Assessment in the Translation Text in LISA QA
The following categories are assessed:
- Mis-translation
- Accuracy - omissions or additions; cross-references; headers and footers
- Terminology - glossary adherence; context
- Language - grammar; semantics; punctuation; spelling
- Style - general style; register and tone; language variants and slang
- Country - country standards; local suitability; company standards
- Consistency
3 Levels of Errors in LISA QA Metric
When using the LISA QA, we can define the severity of errors in 3 levels:
- Critical
- Major
- Minor
As the severity of error increases, the number of points also increase.
- Each minor error found is worth 1 point.
- Each major error found is worth 5 points. This means that one major error is equal to 5 minor errors.
- Critical error is the most serious type of error. Even if only 1 critical error is found, the translation is worthless. The translation fails immediately.
So the maximum error points allowed depends on the number of words in the translated text.
For example, for 1000 words:
- only 6 error points are allowed.
- it can be 6 minor errors
- it can be 1 major error and 1 minor error
Basically the maximum number of error points allowed is proportional to the number of words translated. This proportion is less than 1% of the total number of words.
In the Lisa QA metrics, the numbers of errors are entered in the appropriate fields according to the error category and severity of the error in the software. As the errors are entered, a PASS or a FAIL appears automatically in the “Result” area with green or red color respectively.
The Lisa QA metrics has a user-friendly interface comprising a series of templates, forms and reports embedded in a database (Stejskal 2006):
- a predefined list of error levels of seriousness and relevance
- a record of error categories
- a catalogue of the reviser’s tasks
- a template for marking the translation as Pass or Fail (acceptability threshold).
How can we implement this in Utopian?
For our translation category in Utopian-Davinci collaboration, we encourage translators to do a minimal number of 1000 words. According to the LISA QA metrics, the translator is allowed to make only 6 minor errors to pass the quality of translation.
Since we are still in the process of upgrading the skills of our translators, we can put a cap of allowing 9 minor errors in 1000 word translation. As time goes on, we can bring down the cap to 6 minor errors only. If a translator keeps making high number of errors, we can ask the translator to pause for a while to take some courses to improve on his skill.
As we write out the new guidelines for translators, I believe that it is necessary for language moderators to point out all the errors in the translators and to make note of the frequencies of errors made. The whole point is not to discredit the translator but to evaluate the quality level of the translation work for objective review. In this way, everyone can learn from the review evaluation made by the language moderators.
2. The SAE J2450 metric
SAE J2450 is another metric for measuring translation quality. The tool was first used for revising service automobile documentation so that a consistent quality standard of the automotive service information can be objectively measured. The metrics were not intended for translation where style, register and tone play an important role. Later on with adaptations, the metrics had spread to other industrial sectors such as Biology dealing with pharmaceutical and medical devices.
The SAE tool provides a system for classifying and weighing translation errors in calculating a score for translation quality.
The metrics uses the following category of errors:
1. Wrong Term
- a term is wrong when it violates standard language or industry usage.
- a wrong term is used when it is inconsistent with other translations of the same source term.
2. Syntactic Error
- when words are put incorrect order
- incorrect phrase structure
- misuse of parts of speech
3. Omission & Addition
- An omission occurs when text or a graphic in the source has no counterpart in the translation
- An addition occurs when text or a graphic in the source has no counterpart in the translation
4. Word Structure or Agreement Error
- Error occurs when an otherwise correct target word is expressed in an incorrect morphological form such as case, gender, number, tense, etc.
5. Misspelling
- Different countries may have different spellings for a particular word.
6. Punctuation Error
- The use of wrong punctuation such as commas putting at different places can change the meaning of the text
7. Miscellaneous Error
- Other linguistic error related to the target language which are not clearly attributable to the above categories.
Two Levels of Errors
In the SAE J2450 QA metrics, errors are categorized at 2 levels: Minor or Serious.
For example, if there is one minor and one major misspelling, you would enter "1" in the Minor column and "1" in the Serious column for Misspelling.
When a number is entered in a category, it is multiplied by a weighting figure. For example, under "Misspelling", if there are two minor misspellings, the score would be 2. But if there are 2 serious misspellings, the score would be 6.
Scores for all segments in the task are added together to give a total score for the task. If the overall quality of the task falls below a predefined threshold by the company, the translation work will fail the SAE J2450 check.
This translation metric does not measure errors in style, so it is unsuitable for evaluations of documents where style is important such as owner's manuals or marketing literature.
How can we implement this in Utopian?
This metric seems easier for our present language moderators to implement. There are only 2 levels of severity in the errors: minor and serious. It would be easy to see whether the error is minor or serious.
No software templates are needed for the review. Perhaps, we can draft out a list of error categories for the LM to fill out so as to make their task easier when assigning points to the errors made in the translation.
3. MQM metric
MQM stands for Multidiemensional Quality Metrics. It uses an error typology metric that was developed as part of the (EU-funded) QTLaunchPad project based on careful examination of existing quality models.
This article gives the best description of the MQM-DQF metric:
Multidimensional Quality Metrics (MQM) is a framework for describing and defining custom translation quality metrics. It provides a flexible vocabulary of quality issue types, a mechanism for applying them to generate quality scores, and mappings to other metrics. It does not impose a single metric for all uses, but rather provides a comprehensive catalog of quality issue types, with standardized names and definitions, that can be used to describe particular metrics for specific tasks.
This is the newest metric for error-typology based evaluation.
MQM consists of 20 issues arising in quality assessment of the translated texts. There are 7 major cateogries with subheadings to evaluate the quality of the translation.
1. Accuracy
- mis-translation or ambiguous translation
- addition
- omission
- untranslated
2. Fluency
- Grammar
- Grammatical register
- Inconsistency
- Spelling
- Typography
- Punctuation
- Unintelligible sentences
3. Design
- Incorrect formatting of the document
- Headings are formatted differently from the source
- Broken links
4. Locale Convention
- The text does not adhere to locale-specific mechanical conventions
- The text violates requirements for the presentation of content in the target locale.
- For example, a text translated into Chinese uses Chinese quote marks to indicate titles: 「 and 」.
5. Style
- awkward
- inconsistent
6. Terminology
- The appropriate choice of words
7. Verity
- Completeness
- Legal requirements
- Locale specific content
The MQM metrics can be represented in the following diagram:
Error Count
The MQM scoring metric applies only to error count implementations of MQM. MQM can generate document quality scores according to the following formula:
TQ = 100 − AP − ( FPT − FPS ) − ( VPT − VPS )
TQ = The overall rating of quality Score
AP = Penalties for Accuracy
FPT = Fluency penalties for the target
FPS = Fluency penalties for the source
VPT = Verity penalties for the target
VPS = Verity penalties for the source
The scoring looks quite complex for us as we are not familiar with the system. The lower the score, the higher the translation quality.
How can we implement this MQM metric to Utopian?
To implement this metric, I need to study more in depth regarding the scoring formula. It looks interesting and comprehensive. If we go into more detail work in translation at Utopian, we can consider using this metric.
4. DQF Metric
DQF stands for the Dynamic Quality Framework. Quality is considered "dynamic" because translation quality requirements change all the time depending on the content type, the purpose of the content and its audience.
Therefore, DQF provides a set of tools to evaluate translation quality in its adequacy, fluency, error review, productivity measurement, MT ranking and comparison.
Here is a short description of DQF tools from this article:
DQF provides a vendor independent environment for evaluating translated content. Users can post-edit machine-translated segments to track productivity, evaluate adequacy and fluency of target sentences, compare translations and count errors based on an error-typology. The tools help establish return-on-investment and benchmark performance enabling users to take informed decisions.To ensure evaluation results are reliable it is vital that best practices are applied. The DQF Tools help users apply best practices whether they are selecting their preferred engine, measuring productivity or evaluating the quality of translations.
This chart sums up how DQF works:
The DQF essentially has the same 7 categories as MQM but the subheadings expands a little bit more on the evaluation.
The full DQF evaluation metric is as follows:
1. Accuracy (accuracy)
- Addition
- Improper exact TM match (improper-exact-tm-match)
- Mistranslation (Ambiguous translation; mistranslation of technical relationship; overly literal
- Omission
- Untranslated
2. Design (design)
- Length
- Local formatting
- Markup
- Missing text
- Truncation/text expansion
3. Fluency (fluency)
- Character encoding (character-encoding)
- Grammatical register (grammatical-register)
- Grammar (grammar)
- Inconsistency (Inconsistent with external reference)
- Link/cross-reference (broken-link)
- Punctuation
- Spelling
4. Locale convention
- Address format
- Postal code
- Date format
- Currency format
- Measurement format
- Shortcut key
- Telephone format
5. Style
- Awkward
- Company style
- Inconsistent style
- Third-party style
- Unidiomatic
6. Terminology
- Company terminology
- Third-party termbase
- Inconsistent use of terminology
7. Verity
- the text makes statements that contradict the text
- Culture-specific reference
Despite the variety of approaches taken in industry, the two MQM and DQF metrics turn out to be broadly similar. So developers of MQM and DQF agreed to make changes to both frameworks such that the newly MQM-DQF harmonized metric offers translation professionals a standard and dynamic model that can be used in every context. This is now the newest metric for error-typology based evaluation on translation.
I feel that the translation category in Utopian need to grow into more maturity before we can implement this DQF metric system.
Conclusion
These 4 metric measurements on translation can give our Translation Category a base to work on as we draw out a set of new guidelines and questionnaires to review translation category at Utopian. While many of our verified translators are not at the professional level yet, we can provide guidance to help them improve on their translation quality so that their translations can reach these professional standard metrics.
I have learned so much in this past month on this research on how professionals evaluate a quality translation. Hope you have also benefited much from these articles. I welcome your comments and feedback on this post.
Thank you for your attention,
Rosa
@rosatravels
Blog Post Series
- #1 Promoting Translation in Utopian.io - Fully alive and Stronger
- #2 Promoting Translation in Utopian.io - Growing Translation Teams in Quality
- #3 Promoting Translation in Utopian.io - Growing Performance
- #4 Promoting Translation in Utopian.io - 51 Translators & Proofreaders Getting FOSS projects in Crowdin Go Global
- #5 Translation Category - How To Write Your Application Post to Apply for LM/Translator
- #6 Translation Category Part 1 - What Metrics & Standards Professionals Use To evaluate Translation Quality
- #7 Translation Category Part 2 - Four Standards Professionals Use To evaluate Translation Quality
Thank you for reading this post! If you like the post, please resteem and comment.
谢谢你的阅读!希望你喜欢。如果你喜欢我的分享, 请点赞并跟随我。
.
I continue to be impressed with the depth and detail you are bringing to this task. It is impressive and commendable. I do worry a bit that you'll have a tough time distilling all of this information and knowledge into a usable set of guidelines, but that's a struggle we'll have to deal with at a future date.
Once again, the post had some issues of style and grammar. I see the effort you're making in this area. Keep working at it! I'm happy, as always, to provide examples of issues in a follow-up post.
Your contribution has been evaluated according to Utopian policies and guidelines, as well as a predefined set of questions pertaining to the category.
To view those questions and the relevant answers related to your post, click here.
Need help? Write a ticket on https://support.utopian.io/.
Chat with us on Discord.
[utopian-moderator]
Thanks @didic for your review and feedback. Writing the guidelines and setting specific criteria in the questionnaire will be an uphill struggle. You have so many years of experience behind you so I welcome any suggestions that you have. And regarding grammar correction, yes, by all means share them with me. Thanks for doing all this extra work.
I will definitely be there to help with the guidelines.
Now, on to the examples!
Should be "the translation of the text," or "the translated text," or "the translation itself."
Points are... assigned, maybe?
"Less" should only be used for non count nouns. Basically, if it has a plural form (such as "points"), it should be "fewer," not less.
I think "should have" would work better. Also, on the content side, it's not free from error. Perfection is a goal, not a destination, as even the various metrics you discuss all seem to realize.
The word "of" is redundant. And also, as I just wrote, "error free" is basically impossible. What we want is to minimize errors. Again, every one of the metrics you go on to list supports this.
Awesome @didic. Appreciate this.
Thank you for your review, @didic!
So far this week you've reviewed 14 contributions. Keep up the good work!
This was great work!! Thank you for going into so much depth and detail: it’s a really interesting overview.
These four metric systems all seem like they would really give a boost to our review system. I agree that the DQF seems excessively complicated. At the same time, the Lisa QA is maybe too basic in simply relying on an plain error count, rather than a weighted average like the other methods seem to have.
Maybe we could start by working on a SAE J2450 grid for LMs to use and work towards a MQM as the project (and our collective proficiency) grows.
Thanks @imcesca for your feedback. I agree with you on your observations also. We will try to come up with some specific guideline and criteria for our LM to use so that the review process can be more objective and consistent as the translation category grows.
吃了吗?还在发愁自己的好文没人发现,收益惨淡吗?记得加上cn-curation标签,让飞鸽传书 帮你走出困境吧!倘若你想让我隐形,请回复“取消”。
Congratulations! Your post has been selected as a daily Steemit truffle! It is listed on rank 18 of all contributions awarded today. You can find the TOP DAILY TRUFFLE PICKS HERE.
I upvoted your contribution because to my mind your post is at least 9 SBD worth and should receive 138 votes. It's now up to the lovely Steemit community to make this come true.
I am
TrufflePig
, an Artificial Intelligence Bot that helps minnows and content curators using Machine Learning. If you are curious how I select content, you can find an explanation here!Have a nice day and sincerely yours,
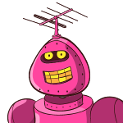
TrufflePig
Hi @rosatravels!
Your post was upvoted by @steem-ua, new Steem dApp, using UserAuthority for algorithmic post curation!
Your UA account score is currently 4.999 which ranks you at #1054 across all Steem accounts.
Your rank has dropped 9 places in the last three days (old rank 1045).
In our last Algorithmic Curation Round, consisting of 304 contributions, your post is ranked at #90.
Evaluation of your UA score:
Feel free to join our @steem-ua Discord server