The Spatial Migration of Political Support
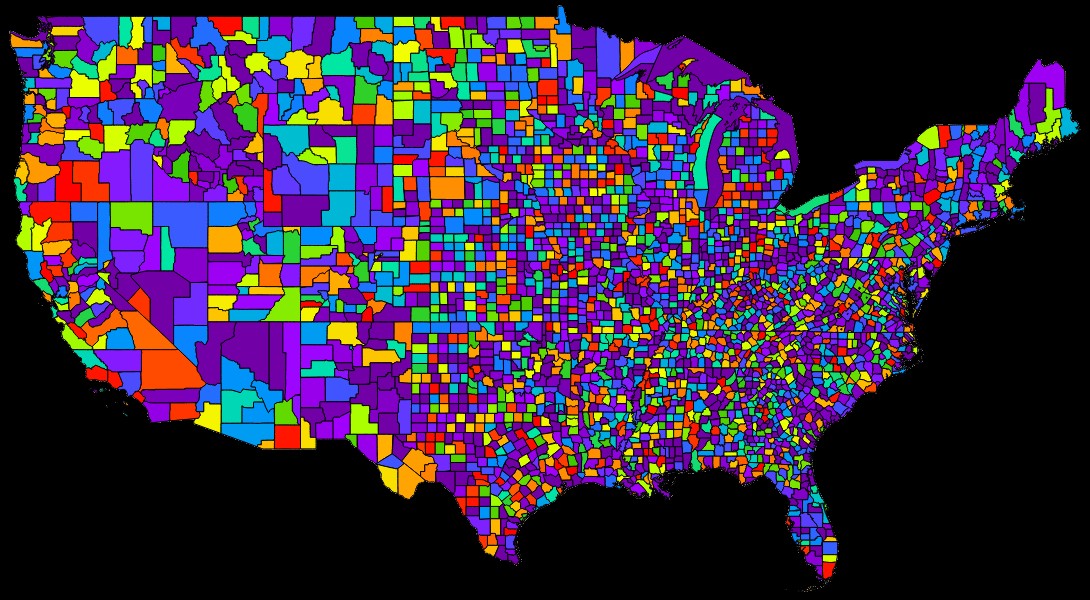
Political Data
The ability to visualize data in a spatial context can be helpful in many disciplines. In previous posts, I have discussed how GIS might be used to hunt for morel mushrooms or Keokuk geodes. Another data type that lends itself to spatial analysis is associated with politics and elections. Elections greatly influence the direction that a country evolves through changes in law. The stakes are so high that billions of dollars were spent during the last presidential election in the United States.Allocating Funds
Knowing where and when to focus efforts in a political campaign can make the difference between winning and losing the election. In the United States, presidential elections are ultimately decided by a process known as the 'Electoral College' rather than by popular vote. There are 538 electors and a majority of 270 electoral votes must be secured to become president. The number of votes that each state provides is based on the number of senators and representatives that the state has, meaning that some states have many electoral votes (California, 55) while others have few (Wyoming, 3).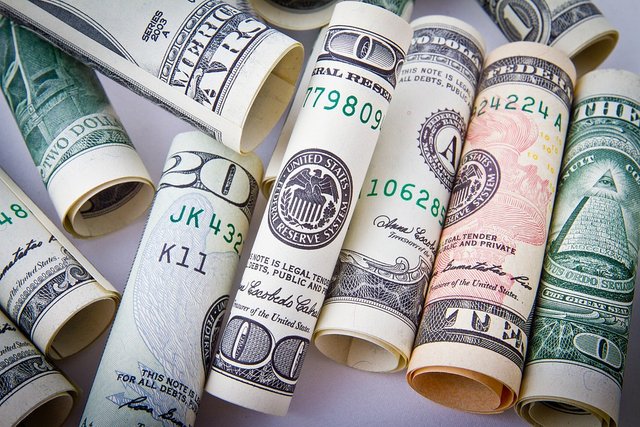
Determining the best way to spend campaign money and the most important places to hold rallies may seem like guesswork, but when election data is visualized spatially and with time there are undeniable trends in the spatial migration of political ideologies that can help inform campaign strategy.
Obtaining the Data
The first step in analyzing election trends is to find spatial data. While state-level data is available from a number of sources, this data is only a coarse average of regional trends. Fortunately there is also county-level data available for the most recent presidential elections that provides much more detail!2004-2012
The 2004, 2008, and 2012 county-level election results have been compiled, summarized, and transformed into a shapefile by the US Federal Government. This data is ready to go and only has to unzipped and loaded into your favorite GIS client.2016
The most recent 2016 election data has not been officially released by the Federal Government. This has not stopped news agencies and data enthusiasts from compiling the county data into a convenient comma delimited file. This file can be joined to a shapefile of US counties to visualize it alongside the 2004-2012 data.2000
Compiled county election data prior to 2004 is difficult to find. I was interested in comparing the 2000 and 2004 elections, but most of the data I found is behind an academic paywall. After a little searching there proved to be another source for the 2000 county data in CSV format. Similar to the 2016 data, this file needs to be joined with a county shapefile to be visualized. In this case, the 2000 data must be edited to account for county boundary changes between 2000 and present day. The main difficulty is in getting the join fields to have the same key, in this case a field called GEOID that is a numerical code representing the state and county. It requires a little more data massaging than the 2016 results, but is the best available source I have found.Visualizing the Data
After the data has been imported into a GIS client and properly projected, the fun begins! For this project I used a WGS 84 projection, but there are many others that work.In QGIS, you can change how the data displays by changing the style in layer properties. I decided to look at the vote percentage and vote count for each party in each of the 5 elections. To view the data this way, choose 'Categorized', style the data based on the preferred column, and stretch to a color ramp of your choosing. The names are mostly self-explanatory and are named by political party (Republican, Democratic) or candidate, but the metadata can be referenced for more details.
Temporal Component
The data that we are attempting to visualize spans 5 elections and 17 years. Because of the temporal component of this project, an animation will make election trends with time easier to spot. To make an animation I take several screenshot images of the different views and import them into an image manipulation program like the free and open-source project GIMP. Here titles can be added and the images can be aligned before exporting the prepared images to a GIF maker. There are several websites and software packages that can generate GIFs, but I often use GifMaker.With animated presentations of election results in hand, the only thing left to do is look for trends! I am not a political theorist and will not analyze the data too deeply, but I'll try to point out a few trends that I notice and let you speculate in the comments!
Democrat Vote Percentage
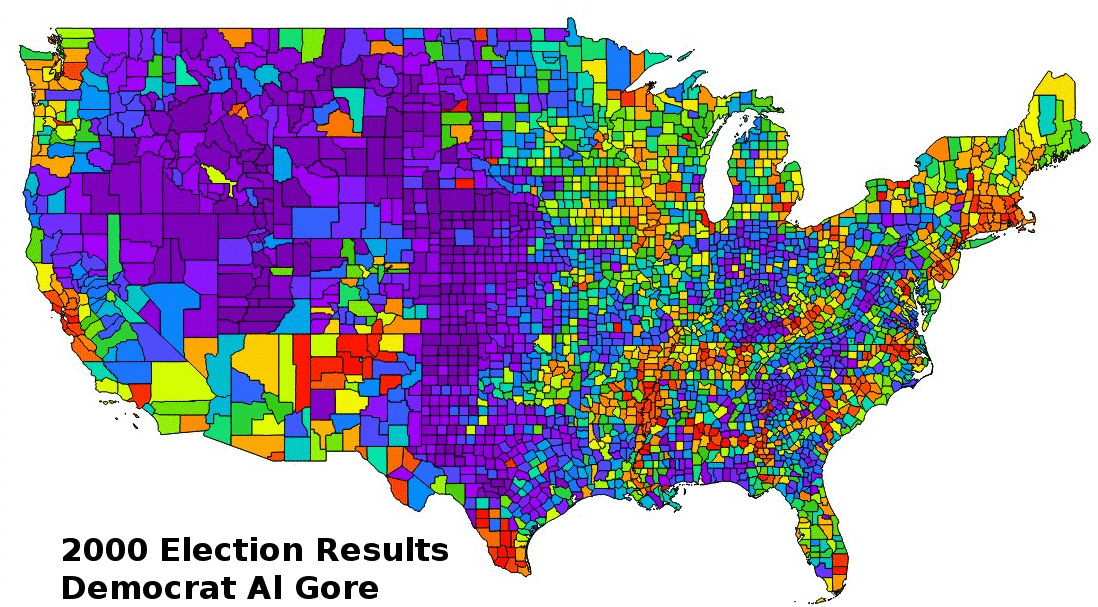
Percentage of votes for the Democratic candidate from 2000-2016. Cooler colors (purples and blues) represent very low percentages, while warmer red and orange colors represent high percentages. Green is generally just less than 50% of the vote and yellow is just over 50%
Republican Vote Percentage
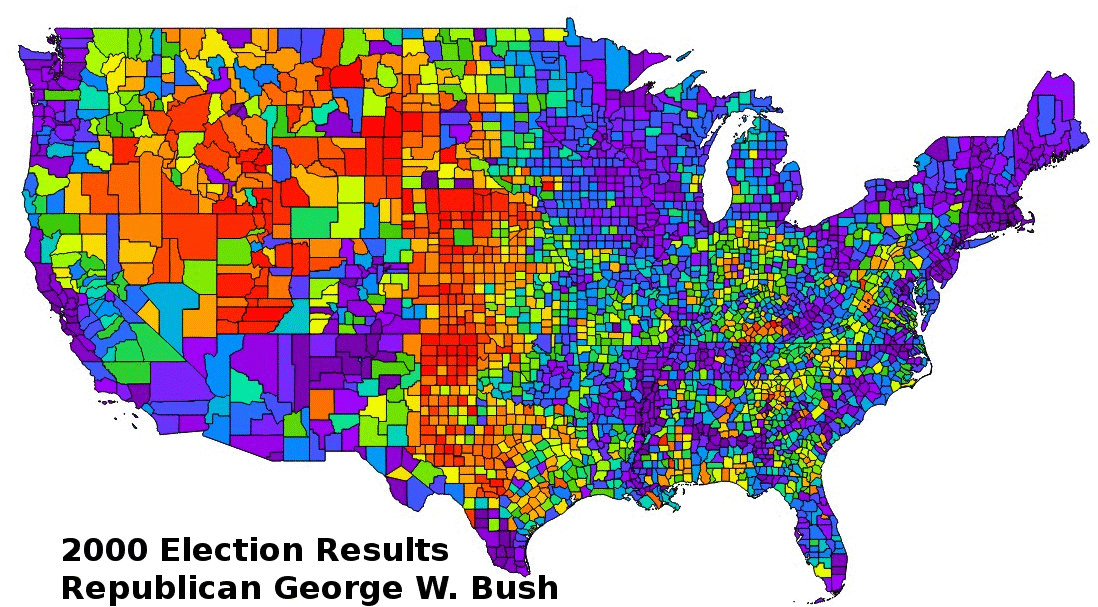
Percentage of votes for the Republican candidate from 2000-2016. Cooler colors (purples and blues) represent very low percentages, while warmer red and orange colors represent high percentages. Green is generally just less than 50% of the vote and yellow is just over 50%
Democrat Vote Count
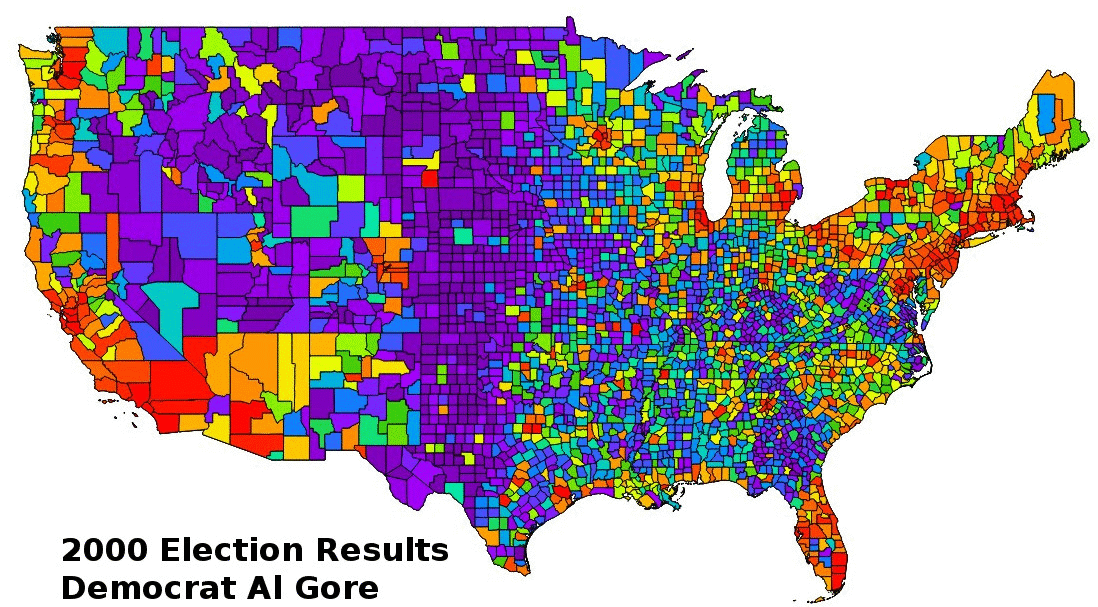
Number of votes for the Democratic candidate from 2000-2016. Cooler colors (purples and blues) represent a small number of votes (10s to 1000s), while warmer red and orange colors represent larger vote counts (100,000s to 1,000,000s).
Republican Vote Count
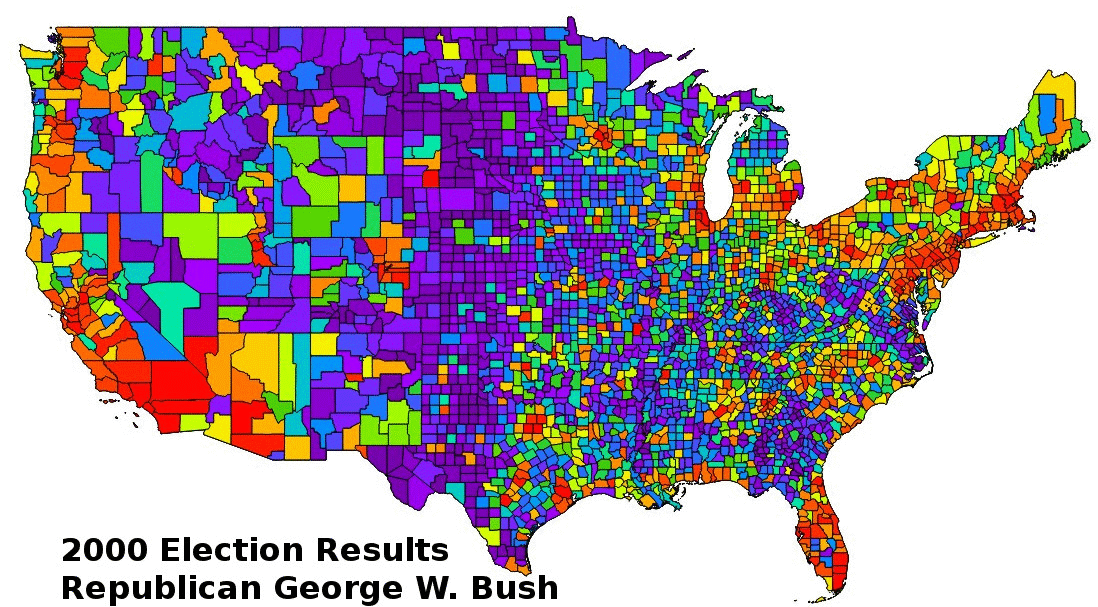
Number of votes for the Republican candidate from 2000-2016. Cooler colors (purples and blues) represent a small number of votes (10s to 1000s), while warmer red and orange colors represent larger vote counts (100,000s to 1,000,000s).
Other Considerations
This analysis is only looking at county level data, not the Electoral College votes that determines presidency. To get a more complete analysis of the different elections, this data should also be considered.Additional trends might be realized if a longer time span is considered. The difficulty is compiling county-level data from historical election data. This requires a lot of effort and until this is done, state-level data is the best available proxy. Other types of information could be extracted from the available county data by combining different fields and running statistical operations.
When importing data, be aware that fields have different data types. If integer values like vote counts are imported as text string, the category style will not sort the fields as expected. An example of the randomness caused by this misake can be seen in the lead photo of this article!
What trends do you see in the data that I did not mention? What other types of data would you like to visualize spatially?
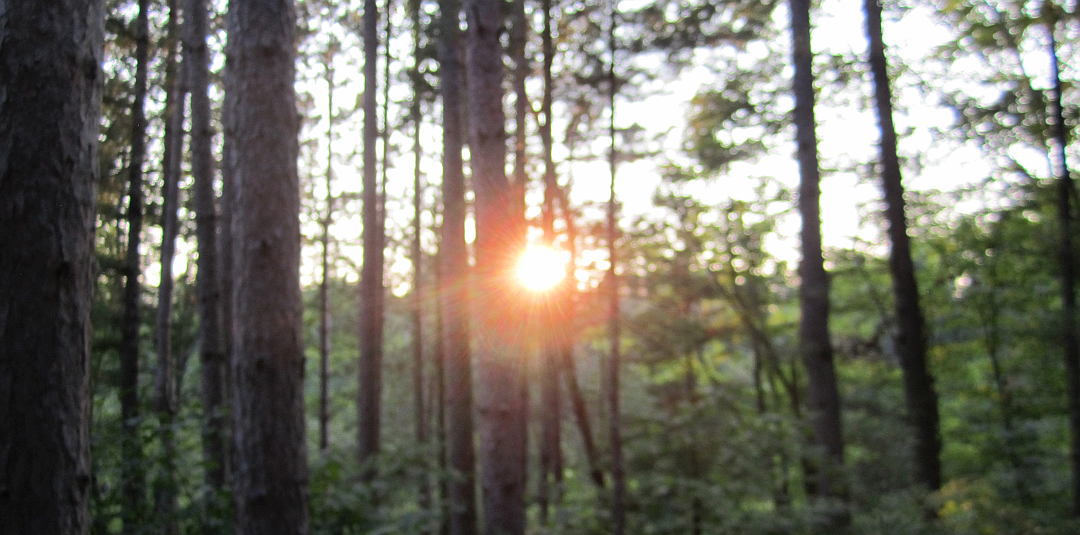
Wow! This is fantastic @gra. This is truly a post showing the power of visualization when looking for trends across massive datasets! I love how you took this very political topic and didn't at all focus on the politics, but rather on the trends! The power of this sort of visualization is it allows you to pick out "buckets" to study further how things changed, and the correlate those changes to what the underlying cause. In the case of this, why are democratc votes so variable while republican votes so very consistent. It likely speaks to the strategies that the political parties utilize for getting out the message to voters and it also explains in part the high degree of success that the republican party has had (as consistency is a key source of knowing what you have, and allows the politicians to better focus on specific areas). When voting support is more variable you can't "count" on any given place and have to spread yourself out much thinner.
Now imagine if voting was done on a blockchain : )
Well hopefully this is something that IS done in the future. Hard to alter votes when they are indelibly written for all to see and trace. Certainly also easier to track the data for these sort of visualizations as well with the information contained.
Yeah and I hope we will see Clinical Trials on the blockchain as well! With the data from smart sensors like from our smartphones to be sent directly to the blockchain. I know output from
Health Kit
andResearch Kit
oniOS
cannot be tampered with.Regardless of where people stand on whether tampering is truly an issue, the blockchain is a superior mechanism over standard servers for this sort of information. Ensuring honestly of reporting should be a focus for everyone, and I am of the opinion that this is the direction we will go in the near future.
I agree, it would be super cool but you know I'm of course worried about special interests and such. So I wonder, ever thought about starting a startup?
I don't know that I possess the necessary technical knowledge with blockchains to function sufficiently to drive a startup to a successful state.
: ) For that you have a CTO : )
I like your assessment of the cause behind the trends @justtryme90, it does seem like the Democratic Party has a more difficult time knowing where to focus their efforts. I also support the idea of getting data of all kinds on a blockchain @nutela, enhancing the transparency and security of the data seems like the only way to move forward.
I just read a paper that kind of pointed to the opposite conclusion: claiming a greater degree of consensus among liberals.
http://journals.sagepub.com/doi/abs/10.1177/1948550617729410
The degree in spatial variability among democratic voters is incredibly interesting here. Maybe it's more of a matter of campaign strategy than underlying values. Really interesting analysis.
A difference in campaign strategy was the point I was trying to make.
Nice work! I only learned about GIS recently when my sister became a geology major, but I'm consistently impressed by its usefulness across a wide range of disciplines. Also, I'm impressed at how you kept this talk out of politics itself and focus on the analysis.
Thanks! I'll have to keep doing different types of GIS articles just to spread the good word and give some other examples! I enjoy the challenge of addressing sensitive subjects in an objective way, though I'm still refining those skills.
Really nice article. I would imagine that this visualization style could be used to show the effects of gerrymandering as well. One could create an algorithm to identify gerrymandered districts (based on their shape), and then only mark those counties with color to identify trends. Theoretically, a gerrymandered county would have no variation (once red, it stays red, and once blue, it stays blue).
This approach could also be interesting to see the effect of local news stations. The Sinclair Broadcast Group has a reputation of inserting right-wing, pro Trump viewpoints into local news programs. It would be interesting to identify the counties covered by these broadcasts and then look at their blue/red coloring over time. For more on Sinclair, see https://www.vox.com/2017/5/15/15598270/sinclair-broadcast-imminent-conservative-takeover-of-local-tv-news-explained.
It would be interesting to try to look at gerrymandering with spatial data. The algorithm would only need to look at the ratio of polygon nodes to polygon area...the gerrymandered districts should show up with a higher number of nodes for a smaller area. I'll have to give it some thought, but I might eventually take a look at the data and see what pops up. Thanks for the thoughts!
You're welcome. There are other algorithms fully covered in computer graphics textbooks (like Graphics Gems) that could be used to identify convex versus concave polygons. Another approach would be to treat it as a physics problem and calculate the center of mass, and then determine how much mass exists in the polygon far from the center. The mean and standard deviation for all county data could then be calculated.
Canada has an independent, non-political commission that makes determinations about gerrymandering, and I remember reading somewhere that they have developed algorithms to do this. If you intend to pursue, it might be useful to track them down and see what techniques they've made publicly available.
It was an eye opener at uni when I discovered that GIS software was not only for mapping and analysing spatial data bit statistical data too. All data cabe mapped. Anazing
I am finding new data that can be plotted and visualized spatially each day! I hope that these posts help others to realize how powerful GIS is!
I have always been in support for a decentralized system for all sorts of spatial data collected and used for electoral processes, be it US or a developing country as mine, Pakistan. A decentralized system would eradicate the possibility of political control by any entity during this important process (elections) in the form of rigging.
It might sound hypothetical and subjective, but I believe no electoral process (developed or under-developed parts of the world) is without the control of institution(s) having high stakes in the outcome of the elections.
I agree, when there is a lot of power and control on the line, some forces will do just about anything to ensure their success. The blockchain as a way to store and protect data from manipulation is going to become more and more common!
Great Post.
Thank you!
@originalworks
The @OriginalWorks bot has determined this post by @gra to be original material and upvoted(2%) it!
To call @OriginalWorks, simply reply to any post with @originalworks or !originalworks in your message!
This post has received a 0.18 % upvote from @drotto thanks to: @gra.