Comparative Characterization of Crofelemer Samples Using Data Mining and Machine Learning Approaches With Analytical Stability Data Sets
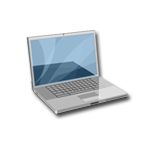
By a News Reporter-Staff News Editor at Information Technology Newsweekly -- Investigators publish new report on Information Technology - Information and Data Mining. According to news reporting out of Santa Fe, New Mexico, by VerticalNews editors, research stated, “There is growing interest in generating physicochemical and biological analytical data sets to compare complex mixture drugs, for example, products from different manufacturers. In this work, we compare various crofelemer samples prepared from a single lot by filtration with varying molecular weight cutoffs combined with incubation for different times at different temperatures.”
Financial support for this research came from Food and Drug Administration.
Our news journalists obtained a quote from the research from Santa Fe Institute, “The 2 preceding articles describe experimental data sets generated from analytical characterization of fractionated and degraded crofelemer samples. In this work, we use data mining techniques such as principal component analysis and mutual information scores to help visualize the data and determine discriminatory regions within these large data sets. The mutual information score identifies chemical signatures that differentiate crofelemer samples. These signatures, in many cases, would likely be missed by traditional data analysis tools. We also found that supervised learning classifiers robustly discriminate samples with around 99% classification accuracy, indicating that mathematical models of these physicochemical data sets are capable of identifying even subtle differences in crofelemer samples.”
According to the news editors, the research concluded: “Data mining and machine learning techniques can thus identify fingerprint-type attributes of complex mixture drugs that may be used for comparative characterization of products.”
For more information on this research see: Comparative Characterization of Crofelemer Samples Using Data Mining and Machine Learning Approaches With Analytical Stability Data Sets. Journal of Pharmaceutical Sciences , 2017;106(11):3270-3279. Journal of Pharmaceutical Sciences can be contacted at: Wiley, 111 River St, Hoboken 07030-5774, NJ, USA. (Wiley-Blackwell - http://www.wiley.com/; Journal of Pharmaceutical Sciences - http://onlinelibrary.wiley.com/journal/10.1002/(ISSN)1520-6017)
Our news journalists report that additional information may be obtained by contacting E.J. Deeds, Santa Fe Inst, Santa Fe, NM 87501, United States. Additional authors for this research include J.H. Kim, J. Xiong, P.A. Kleindl, A. Hewarathna, A.C. Fisher, S.B. Joshi, C. Schoneich, M.L. Forrest, C.R. Middaugh, D.B. Volkin and M.K. Nariya.
The direct object identifier (DOI) for that additional information is: https://doi.org/10.1016/j.xphs.2017.07.013. This DOI is a link to an online electronic document that is either free or for purchase, and can be your direct source for a journal article and its citation.
Our reports deliver fact-based news of research and discoveries from around the world. Copyright 2018, NewsRx LLC
CITATION: (2018-01-09), Investigators from Santa Fe Institute Report New Data on Information and Data Mining (Comparative Characterization of Crofelemer Samples Using Data Mining and Machine Learning Approaches With Analytical Stability Data Sets), Information Technology Newsweekly, 76, ISSN: 1944-1800, BUTTER® ID: 014954482
From the newsletter Information Technology Newsweekly.
https://www.newsrx.com/Butter/#!Search:a=14954482
NewsRx® offers 195 weekly newsletters providing comprehensive information on all professional topics, ranging from health, pharma and life science to business, tech, energy, law, and finance. Our newsletters report only the most relevant and authoritative information from qualified sources.